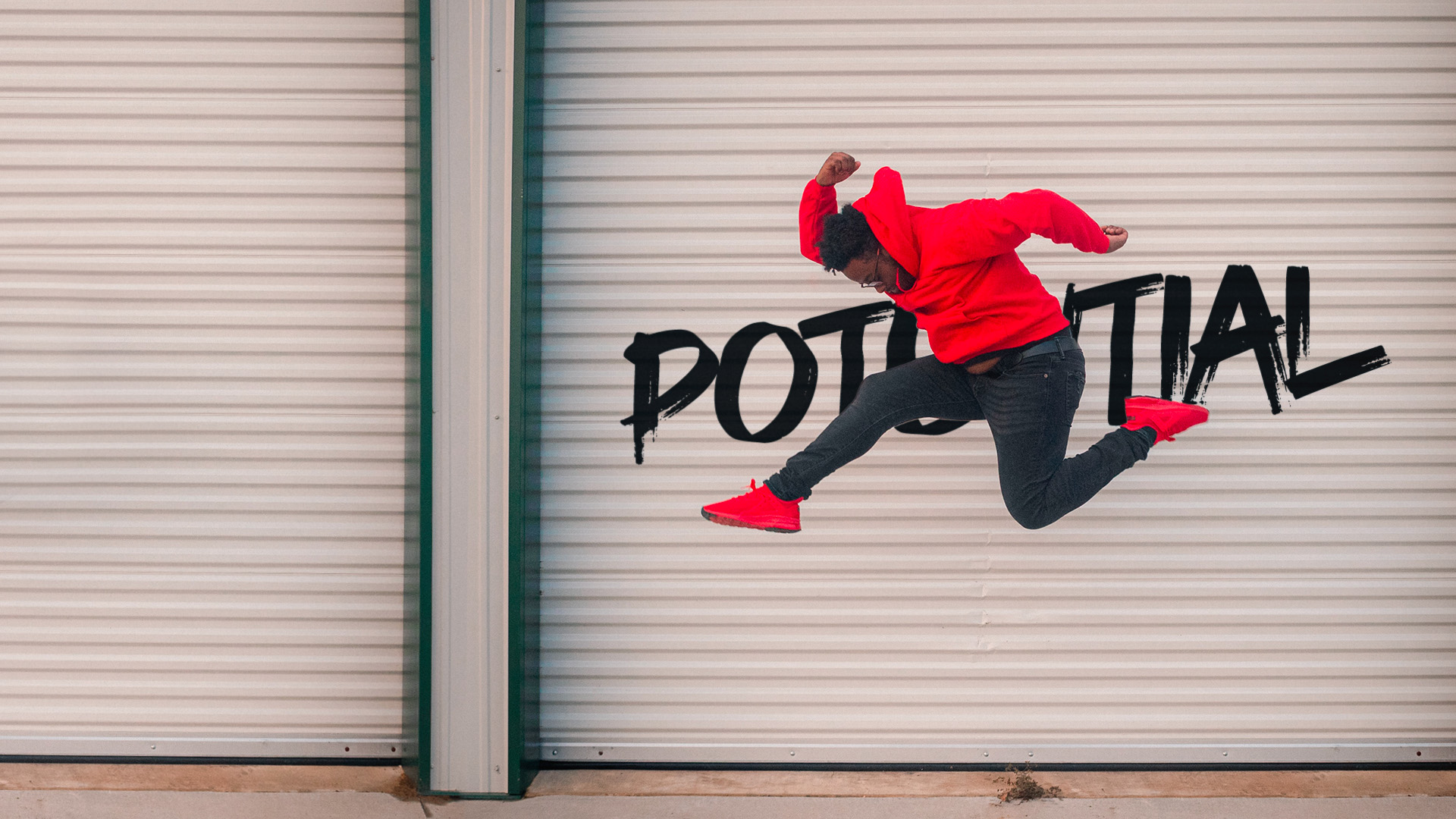)
Unleash the true potential of Quantum Machine Learning
What makes Quantum Computing so interesting
In recent years, quantum computing has grown in various industries, including finance, healthcare, telecommunications, cybersecurity, and logistics. Its widespread interest stems from its unique computational capabilities and potential advantages over classical computing.
Industries face complex computational challenges, especially in process optimization and decision-making. Quantum computing, utilizing effects like superposition and entanglement, promises faster solutions than classical computing. While machine learning has seen successes, it often requires substantial data and resources, sometimes leading to suboptimal results or limitations in applying ML at business scales. The synergy between quantum computing and machine learning is emerging, with quantum seen as a way to enhance ML solutions, either by faster model training or better data pattern recognition.
Your quantum future begins now
Quantum Machine Learning (QML) merges quantum computing and machine learning, aiming to enhance computing speed and precision. By integrating classical computer stability with quantum effects like superposition and entanglement, hybrid approaches like variational algorithms show potential in overcoming hardware noise and decoherence challenges. These algorithms work well with near-term NISQ devices, offering improved solutions for machine learning and optimization.
Modern quantum development libraries, compatible with standard programming languages, facilitate quantum circuit testing and work on various platforms, including the cloud. While the QML field is rapidly advancing with contributions from academia, effectively applying it in business requires expertise, as benchmarks and optimal combinations of tools are still evolving.
Reply’s Experience
At Reply, we have gained extensive experience in implementing and benchmarking different quantum algorithms, and our experts in quantum technologies can recommend which algorithms and systems to use when addressing one of your use cases. Don't miss your chance to join the race and explore with us the best quantum solutions for your needs.